CBI partnered with eastnets to strengthen sanctions screening
- Resources
- Case studies
- CBI partnered with eastnets to strengthen sanctions screening
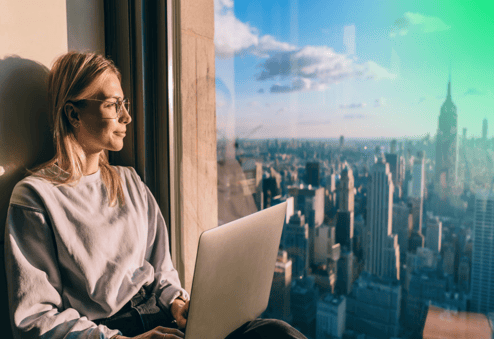
As a local leading bank recognized throughout the UAE, CBI focuses on digitization and the human element to constantly improve its operations and customer service standards.
Through a suite of Eastnets’ easyto-integrate products, the bank can further improve detection rates, reduce infrastructure burdens, and quickly adapt to regulatory changes, enabling it to continue its focus on delivering superior services to its customers.
About CBI
CBI has been helping leading companies and ambitious individuals in the UAE manage their business and personal finances with confidence since 1991. The bank’s mission is to serve its customers by helping them prosper in both their personal and professional lives. CBI is committed to excellence and leadership, continually investing in the latest technology and developing a deeper personal understanding of their customers.
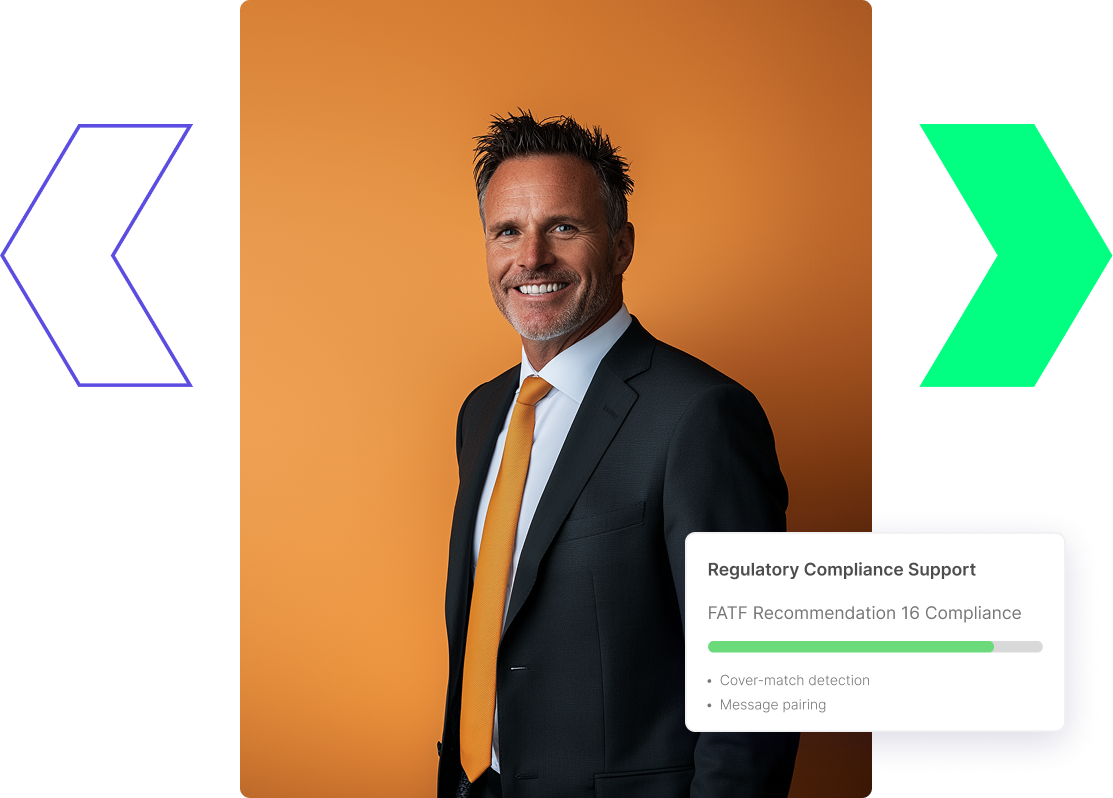
Solution
Eastnets action plan
The solution provides fuzzy matching watchlist screening capabilities for real-time transactions, information batch mode capabilities, and other ad-hoc screening requirements.
Using SafeWatch Screening, CBI reduces false positives such as matching scores, good guys and violation/ business rules. The bank can continuously monitor all inbound and outbound customer information and financial messaging, including newly onboarded customers. With the solution’s automatic updates feature, CBI can also adhere to new regulations even more rapidly than before.
Additionally, using dual control and advanced system workflows, CBI can escalate suspicious activities to higher levels of management for special investigations with improved speed.
-
PaymentSafe.
With PaymentSafe, CBI can quickly, securely, and cost-effectively centralize the Straight-ThroughProcessing (STP) of multiple financial workflows. The bank is also able to respond to new regulations and messaging formats with improved efficiency.
-
SWIFT Hosting
The solution enables CBI to offload all SWIFT-related activities and eliminate the challenge of maintaining and managing the infrastructure behind it; in turn, driving down costs while also increasing service reliability. CBI also leverages the solution’s duplicate message detection functionality to help minimize the operational issues, expenses and processes around reconciliation.
-
SafeWatch Screening
SafeWatch Screening is a SWIFT certified product for advanced sanction and watchlist screening that has been installed in over 360 banks worldwide and granted the SWIFT Alliance Plug-in label for its seamless integration with the SWIFT environment.
Results
Improved detection rates
Real-time transaction filtering capabilities.
Products used
SafeWatch Screening
SWIFT Hosting
PaymentSafe
Mr Walid Elmasri CBI Head of Technology
The next products in your Anti Money Laundering journey
Eastnets products are toolsets designed to work together for maximum protection and security. Stacking our products into a modular solution that fits your exact needs is highly recommended to reduce financial crime, fraud and other nefarious acts that could compromise your network.
Arrange a demo
Our fraud and compliance experts are ready to help build you a custom solution. Book a demo with our team today and start your journey to compliance bliss.
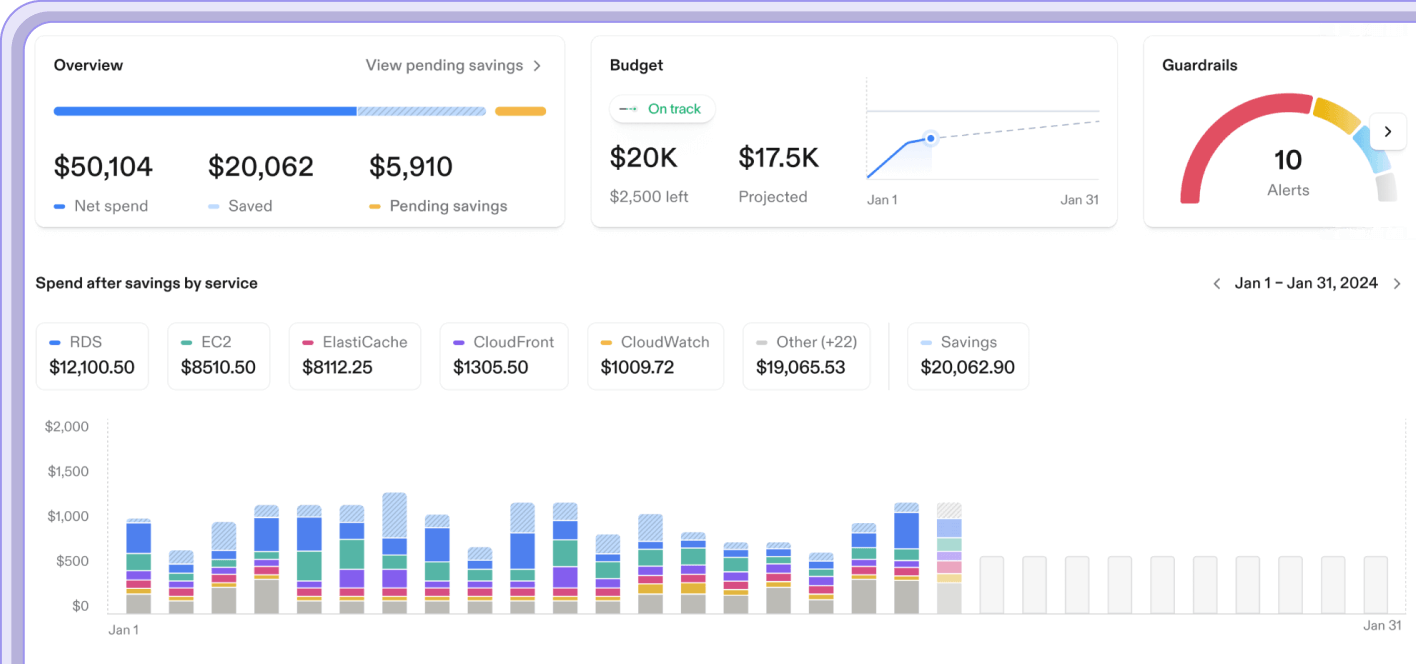